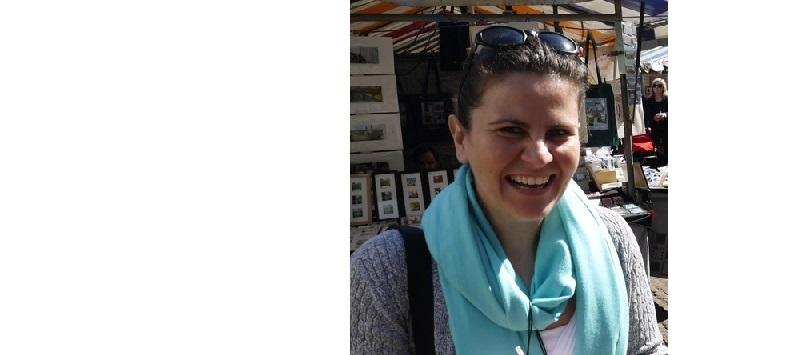
LEVERHULME EARLY CAREER FELLOW SUPPORTED BY THE ISAAC NEWTON TRUST
UCL
I am interested in understanding the complex networks of interactions at the cellular level as well as those across species, and how these translate into a system-level understanding of organisms, populations or mixed-communities, which can then be exploited for biotechnological applications. A systems-based analysis integrating functional genomics and metabolomics with theoretical models allows us to seek a deeper understanding of how functional entities and modules facilitate the flow of biological information at the cellular level as well as across single-cell species. My work therefore follows three main streams of interest; (i) systems based understanding and exploitation of organisms through integration of “omics” technologies and network- / model-based analysis, (ii) optimisation and predictive-analysis of upstream processing of cell and microbial cultures in biotechnology applications, and (iii) development of methodologies and pipelines to assist these goals.
Research areas:
Systems Biology and Biotechnology
Model based analysis of biological systems
Bioprocess engineering and upstream process development for biomanufacturing pharmaceuticals